Generating brain-optimized image for advancing research efficiency
Generating brain-optimized image for advancing research efficiency
Stanford University
2024
One of the fundamental goals of neuroscience is to
understand how stimulus information is transformed into neuronal responses. To
create a computational model that captures this transformation, it is necessary
to collect neuronal responses across a diverse range of stimuli. However, due
to the extensive range of possible stimuli, it is challenging to only select an
optimal set of stimuli that can effectively elicit significant neural
modulations. Moreover, there are practical limitations on the number of
measurements and the duration of experiments that experimenters can perform at
each time. As a result, experimenters rely heavily on their own prior knowledge
to elicit the desired neural responses. However, this approach may not always result in an
optimal experiment tailored to address their specific research questions.
To tackle this problem, I am currently developing a novel software tool that generates an optimal set of stimuli, tailored to the specific hypotheses of each researcher. Specifically, I am leveraging recent advancements in generative deep neural networks, such as GANs and stable diffusion models, to generate stimuli in a manner directly optimized to the brain response itself. The goal is to produce a set of stimuli that would maximize the performance of a brain model, which, in turn, would most effectively modulate underlying neural responses.
To tackle this problem, I am currently developing a novel software tool that generates an optimal set of stimuli, tailored to the specific hypotheses of each researcher. Specifically, I am leveraging recent advancements in generative deep neural networks, such as GANs and stable diffusion models, to generate stimuli in a manner directly optimized to the brain response itself. The goal is to produce a set of stimuli that would maximize the performance of a brain model, which, in turn, would most effectively modulate underlying neural responses.

Examples of brain optimized images:
Images optimized for early visual area (V1)
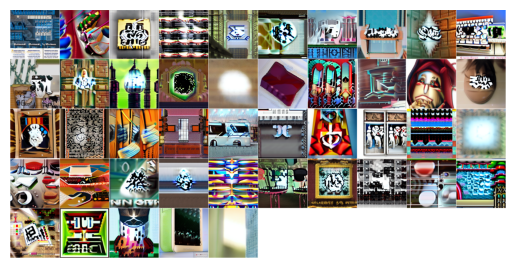
Images optimized for fusiform face area (FFA)
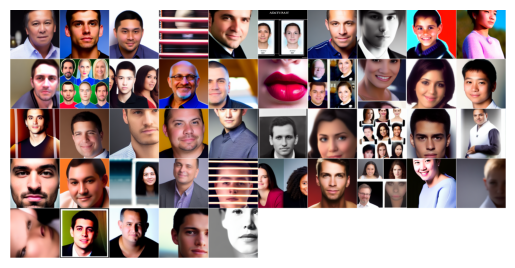
Images optimized for parahippocampal place area (PPA)
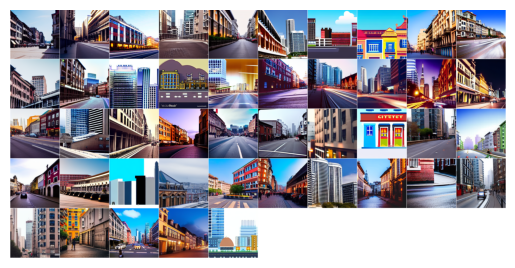
Testing pRF recovery profile with natrualistic images
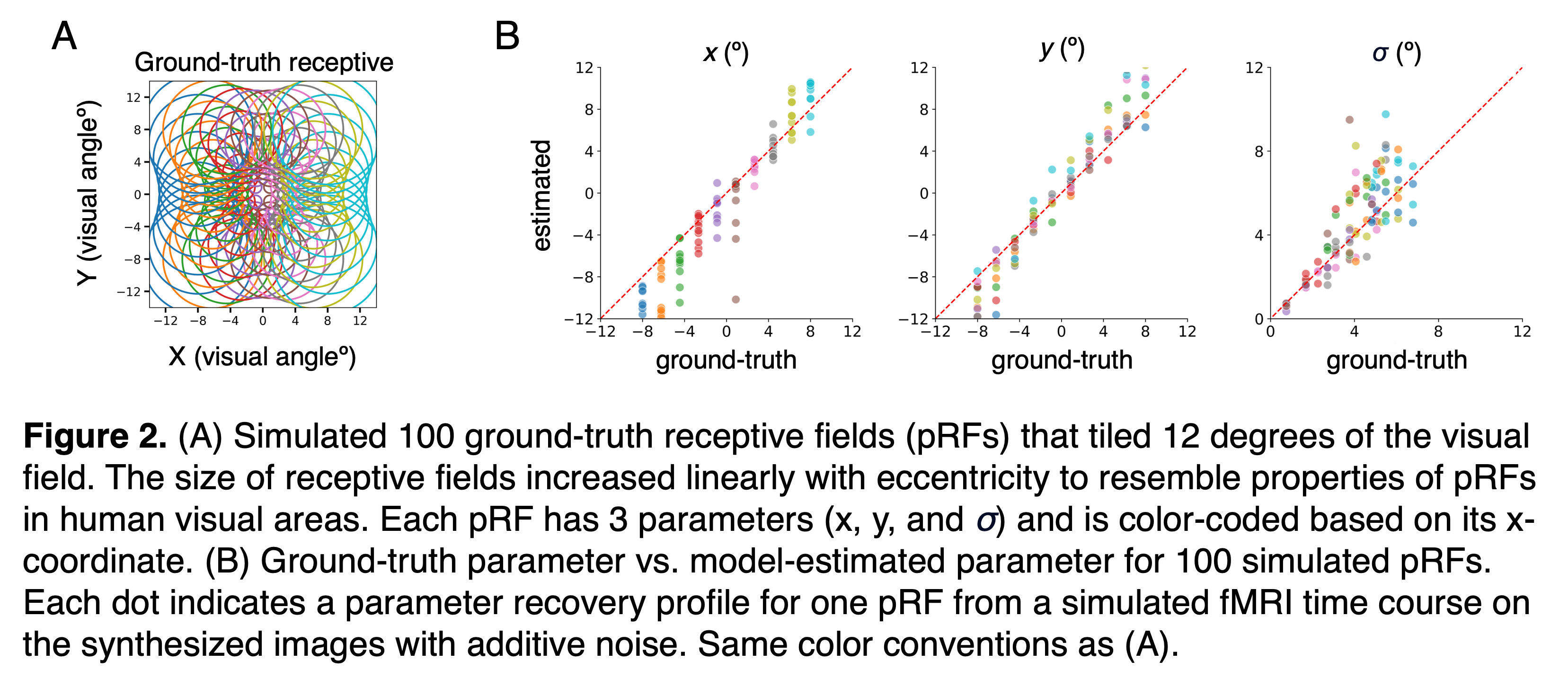